EvalRS Keynote
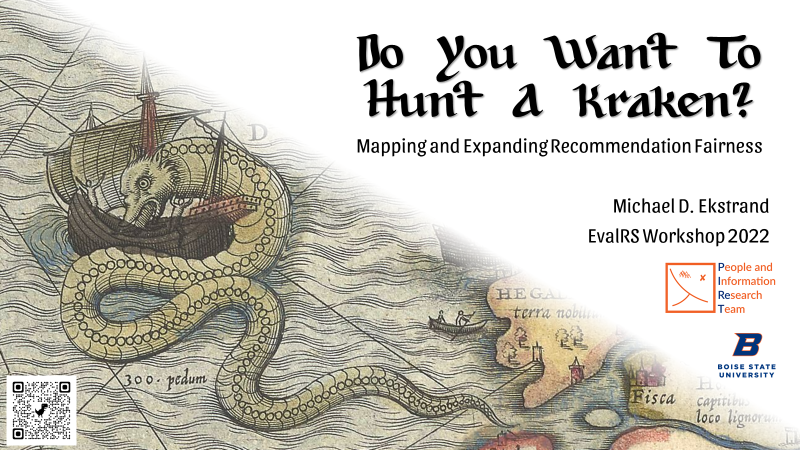
I will be giving a keynote talk, “Do You Want To Hunt A Kraken? Mapping and Expanding Recommendation Fairness”, at the EvalRS workshop at CIKM 2022.
The video is now available. Fair recommendation (and related problems, such as fair information retrieval) is a complex, multi-faceted problem. Significant progress has been made in recent years on identifying and measuring important forms of unfair recommendation, but there are still many ways recommender systems can replicate, exacerbate, or mitigate potentially discriminatory harms that need careful study. In this talk, I will provide an overview of the landscape of fairness and anti-discrimination in information access systems, discussing both the state of the art in measuring relatively well-understood harms and new directions and open problems in defining and measuring fairness problems. This will set the workshop’s metrics and objectives in a broad context and hopefully catalyze discussion about what the next iteration of EasyRS and research following up on conference outcomes might look like. The maps are from the Carta marina et descriptio septentrionalium terrarum by Olaus Magnus (1539), redrawn by Antoine Lafréry in 1572. I discuss the following papers in this talk: 2022. Fairness in Information Access Systems. Foundations and Trends® in Information Retrieval 16(1–2) (July 2022), 1–177. DOI 10.1561/1500000079. arXiv:2105.05779 [cs.IR]. NSF PAR 10347630. Impact factor: 8. Cited 205 times. Cited 97 times. 2022. Matching Consumer Fairness Objectives & Strategies for RecSys. Presented at the 5th FAccTrec Workshop on Responsible Recommendation at RecSys 2022 (peer-reviewed but not archived). arXiv:2209.02662 [cs.IR]. Cited 5 times. Cited 3 times. 2022. Measuring Fairness in Ranked Results: An Analytical and Empirical Comparison. In Proceedings of the 45th International ACM SIGIR Conference on Research and Development in Information Retrieval (SIGIR ’22), Jul 11, 2022. pp. 726–736. DOI 10.1145/3477495.3532018. NSF PAR 10329880. Acceptance rate: 20%. Cited 73 times. Cited 51 times. 2021. Exploring Author Gender in Book Rating and Recommendation. User Modeling and User-Adapted Interaction 31(3) (February 2021), 377–420. DOI 10.1007/s11257-020-09284-2. arXiv:1808.07586v2. NSF PAR 10218853. Impact factor: 4.412. Cited 206 times (shared with RecSys18◊). Cited 112 times (shared with RecSys18◊). 2022. Fire Dragon and Unicorn Princess: Gender Stereotypes and Children’s Products in Search Engine Responses. In SIGIR eCom ’22, Jul 15, 2022. 9 pp. DOI 10.48550/arXiv.2206.13747. arXiv:2206.13747 [cs.IR]. Cited 14 times. Cited 6 times. Other research cited:Abstract
Slides
My Research
Citations