Nov. 2022 talk at Waseda University
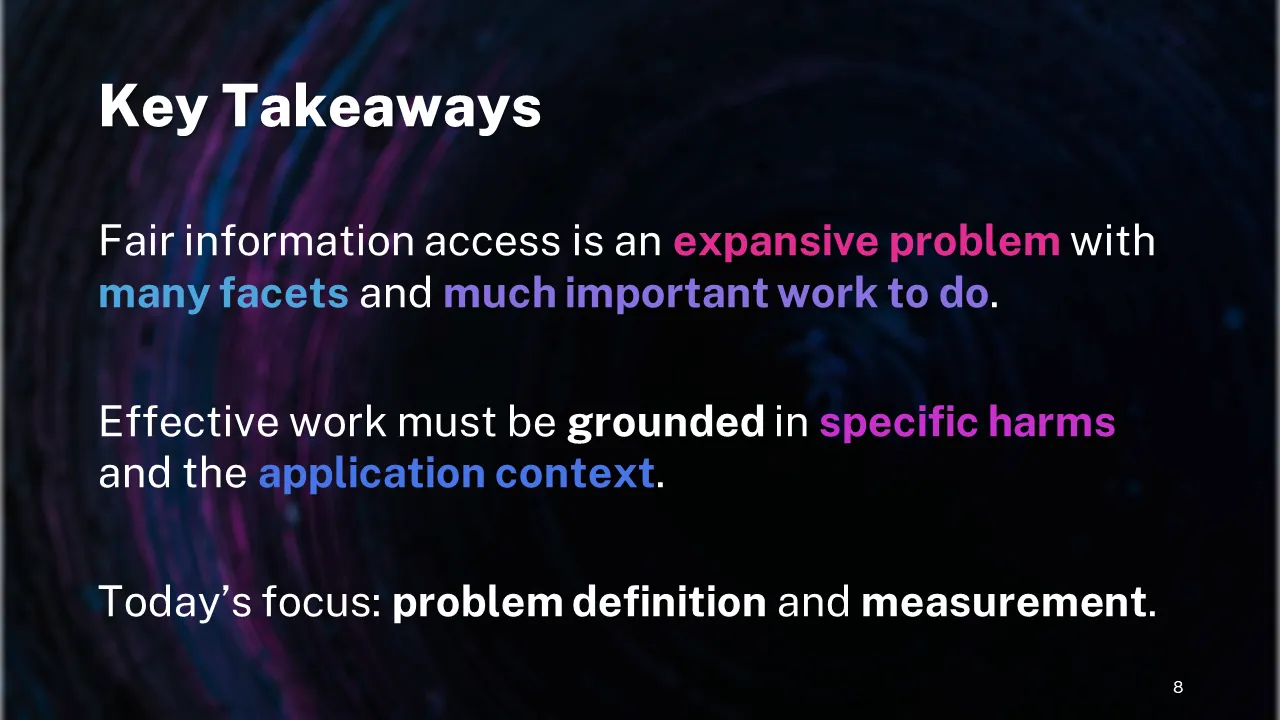
I will be giving a seminar talk, “Equity and Discrimination in Information Access”, at Waseda University on Nov. 19, 2022. Ensuring that information access systems are “fair”, or that their benefits are equitably experienced by everyone they affect, is a complex, multi-faceted problem. Significant progress has been made in recent years on identifying and measuring important forms of unfair recommendation and retrieval, but there are still many ways that information systems can replicate, exacerbate, or mitigate potentially discriminatory harms that need careful study. These harms can affect different stakeholders — such as the producers and consumers of information, among others — in many different ways, including denying them access to the system’s benefits, misrepresenting them, or reinforcing unhelpful stereotypes. In this talk, I will provide an overview of the landscape of fairness and anti-discrimination in information access systems, discussing both the state of the art in measuring relatively well-understood harms and new directions and open problems in defining and measuring fairness problems. This talk will draw heavily from our paper: 2022. Fairness in Information Access Systems. Foundations and Trends® in Information Retrieval 16(1–2) (July 2022), 1–177. DOI 10.1561/1500000079. arXiv:2105.05779 [cs.IR]. NSF PAR 10347630. Impact factor: 8. Cited 207 times. Cited 97 times. This paper is available free (with registration) from doi:10.1561/1500000079 from Nov. 17–21. This talk also discusses the following work we have published: 2022. Matching Consumer Fairness Objectives & Strategies for RecSys. Presented at the 5th FAccTrec Workshop on Responsible Recommendation at RecSys 2022 (peer-reviewed but not archived). arXiv:2209.02662 [cs.IR]. Cited 5 times. Cited 3 times. 2022. Fire Dragon and Unicorn Princess: Gender Stereotypes and Children’s Products in Search Engine Responses. In SIGIR eCom ’22, Jul 15, 2022. 9 pp. DOI 10.48550/arXiv.2206.13747. arXiv:2206.13747 [cs.IR]. Cited 14 times. Cited 6 times. 2022. Measuring Fairness in Ranked Results: An Analytical and Empirical Comparison. In Proceedings of the 45th International ACM SIGIR Conference on Research and Development in Information Retrieval (SIGIR ’22), Jul 11, 2022. pp. 726–736. DOI 10.1145/3477495.3532018. NSF PAR 10329880. Acceptance rate: 20%. Cited 74 times. Cited 52 times. 2022. The Multisided Complexity of Fairness in Recommender Systems. AI Magazine 43(2) (June 2022), 164–176. DOI 10.1002/aaai.12054. NSF PAR 10334796. Cited 39 times. Cited 21 times. 2021. Exploring Author Gender in Book Rating and Recommendation. User Modeling and User-Adapted Interaction 31(3) (February 2021), 377–420. DOI 10.1007/s11257-020-09284-2. arXiv:1808.07586v2. NSF PAR 10218853. Impact factor: 4.412. Cited 209 times (shared with RecSys18. Cited 114 times (shared with RecSys18. 2020. Evaluating Stochastic Rankings with Expected Exposure. In Proceedings of the 29th ACM International Conference on Information and Knowledge Management (CIKM ’20), Oct 21, 2020. ACM, pp. 275–284. DOI 10.1145/3340531.3411962. arXiv:2004.13157 [cs.IR]. NSF PAR 10199451. Acceptance rate: 20%. Nominated for Best Long Paper. Cited 200 times. Cited 175 times.Abstract
Slides
Resources
Work Cited